A promising algorithm for early diagnosis of Acid Sphingomyelinase Deficiency (ASMD) in patients with unexplained Interstitial Lung Disease (ILD). Leveraging Electronic Health Records (EHR) and machine learning, a ASMD diagnostic algorithm, was developed achieving remarkable results. Indeed, it distinguished ASMD patients from a general population with a sensitivity of 80% and a specificity of over 99%. When applied to an ILD unexplained cohort, the algorithm flagged 691 potential ASMD cases.
Pharmaceutical companies specialized in rare diseases should take note of this innovation’s potential. Such an algorithm could help primary care practitioners and specialists in screening patients potentially undiagnosed with ASMD but also, as it is interpretable, generate new medical hypotheses on the natural history of the disease. While further validation is required, the prospects are promising.
The convergence of machine learning and healthcare is transforming personalized medicine, offering hope for those with rare diseases. Let’s continue pushing boundaries against diseases !
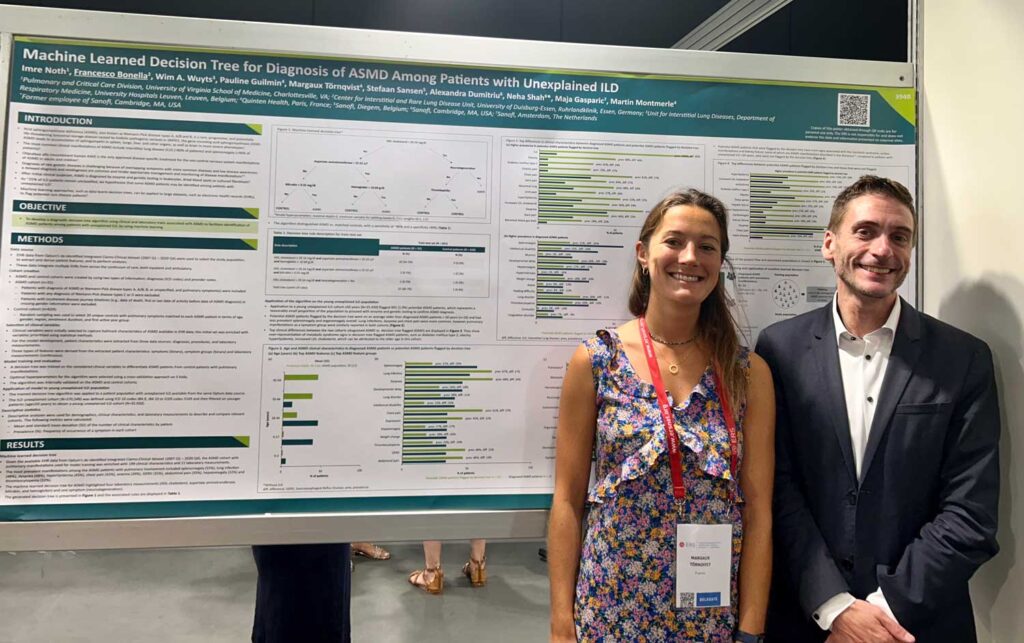
Margaux Törnqvist and Francesco Bonella at the annual European Respiratory Society congress 2023 in Milan.
Quinten Health’s team presented this work with the poster “Machine learned decision tree for diagnosis of ASMD among patients with unexplained ILD” at the annual European Respiratory Society congress in Milan.
Margaux Törnqvist represented the team during the poster presentation session entitled “New approaches in rare interstitial lung diseases”.
Pauline Guilmin was also an author of this poster alongside Imre Noth, Francesco Bonella, Wim A. Wuyts, Stefaan Sansen, Alexandra Dumitriu, Neha Shah, Maja Gasparic and Martin Montmerle.